Research
Information Theory
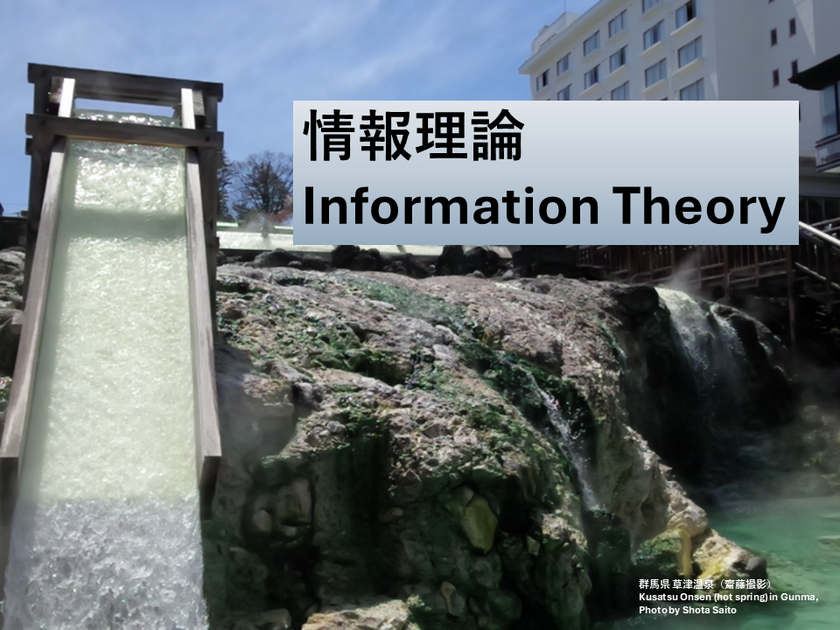
Lossy Source Coding
Example of Research
We investigated variable-length lossy source coding with the criteria of the normalized cumulant generating function of codeword lengths and the excess distortion probability, and analyzed the non-asymptotic fundamental limit of the normalized cumulant generating function of codeword lengths under the constraint that the excess distortion probability is allowed up to $\epsilon \in [0,1)$. The results of this research have been published in the IEEE Transactions on Information Theory.
S. Saito and T. Matsushima, "Non-Asymptotic Bounds of Cumulant Generating Function of Codeword Lengths in Variable-Length Lossy Compression," in IEEE Transactions on Information Theory, vol. 69, no. 4, pp. 2113-2119, April 2023, doi: 10.1109/TIT.2022.3229358. Link
Lossless Source Coding
Bayes code
A Bayes code is one of the universal lossless source code.
Example of Research 1)
We proved that a Bayes code has been theoretically shown to exhibit “good” performance under a variety of evaluation criteria.
S. Saito and T. Matsushima, "Evaluation of Overflow Probability of Bayes Code in Moderate Deviation Regime," in IEICE Transactions on Fundamentals of Electronics, Communications and Computer Sciences, vol. E100-A, no. 12, pp. 2728-2731, December 2017, doi: 10.1587/transfun.E100.A.2728. Link
S. Saito, N. Miya and T. Matsushima, "Evaluation of the Bayes Code from Viewpoints of the Distribution of Its Codeword Lengths," in IEICE Transactions on Fundamentals of Electronics, Communications and Computer Sciences, vol. E98-A, no. 12, pp. 2407-2414, December 2015, doi: 10.1587/transfun.E98.A.2407. Link
S. Saito, N. Miya and T. Matsushima, "Fundamental limit and pointwise asymptotics of the Bayes code for Markov sources," 2015 IEEE International Symposium on Information Theory (ISIT), Hong Kong, China, 2015, pp. 1986-1990, doi: 10.1109/ISIT.2015.7282803. Link
Example of Research 2)
We developed an efficient Bayes coding algorithm for non-stationary source where a context tree source changes.
K. Shimada, S. Saito and T. Matsushima, "An Efficient Bayes Coding Algorithm for Changing Context Tree Model," in IEICE Transactions on Fundamentals of Electronics, Communications and Computer Sciences, vol. E107.A, no. 3, pp. 448-457, March 2024, doi: 10.1587/transfun.2023TAP0017. Link
Smooth-max entropy
Example of Research 1)
S. Saito and T. Matsushima, "Threshold of Overflow Probability Using Smooth Max-Entropy in Lossless Fixed-to-Variable Length Source Coding for General Sources," in IEICE Transactions on Fundamentals of Electronics, Communications and Computer Sciences, vol. E99-A, no. 12, pp. 2286-2290, December 2016, doi: 10.1587/transfun.E99.A.2286. Link
Example of Research 2)
S. Saito and T. Matsushima, "Second-Order Achievable Rate Region of Slepian-Wolf Coding Problem in terms of Smooth Max-Entropy for General Sources," in IEICE Transactions on Fundamentals of Electronics, Communications and Computer Sciences, vol. E99.A, no. 12, pp. 2275-2280, December 2016, doi: 10.1587/transfun.E99.A.2275. Link
Guessing
Example of Research
S. Saito, "Soft Guessing Under Log-Loss Distortion Allowing Errors," IEEE International Symposium on Information Theory (ISIT), 2024.